Predictive maintenance at scale
Embrace predictive maintenance across all assets and sites to boost productivity, sustainability, and digital transformation. Predictive Maintenance directly addresses key manufacturer challenges, minimizing downtime and optimizing resource allocation for maximum efficiency and growth.
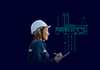
Embrace predictive maintenance across all assets and sites to boost productivity, sustainability, and digital transformation. Predictive Maintenance directly addresses key manufacturer challenges, minimizing downtime and optimizing resource allocation for maximum efficiency and growth.